Unlock efficiency and profitability with predictive analytics
EPA System / News & Eventi
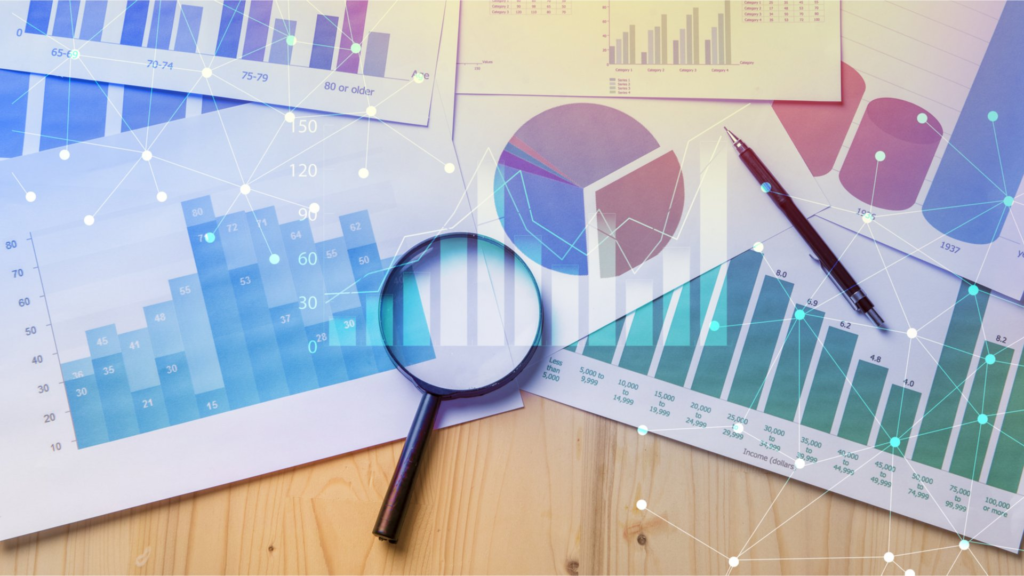
Energy is a valuable and costly resource, and companies in the sector are constantly seeking ways to optimize their operations to reduce costs and maximize profits. Predictive analysis offers an innovative way to achieve these goals, using algorithms and mathematical models to predict future behaviors and make informed decisions.
The importance of efficiency and profitability in energy operations
Efficiency and profitability are two critical factors for the success of energy operations. The energy industry is characterized by intense competition and narrow profit margins. Therefore, it is essential for companies to find ways to improve the efficiency of their operations to reduce costs and increase profitability. Predictive analysis provides a unique opportunity to gain competitive advantages by allowing companies to make data-driven decisions that can improve the efficiency of their operations and maximize profits.
Understanding predictive analysis and its benefits
Predictive analysis is a technique that uses historical data and mathematical models to make predictions about the future. In the context of energy operations, predictive analysis can be used to predict energy demand, identify consumption patterns, anticipate machinery failures, and optimize energy production. This enables companies to make informed decisions and anticipate potential issues, reducing operational costs and improving overall productivity.
Key considerations for implementing predictive analysis in the energy sector
Before implementing predictive analysis in the energy sector, there are several key considerations to keep in mind. Firstly, it is important to have a solid and scalable IT infrastructure to support the processing of large amounts of data and the execution of complex analytical models. Secondly, it is necessary to collect and integrate data from various sources, such as sensors, monitoring systems, and historical data. Finally, it is crucial to have teams of experts in data analysis and expertise in the energy sector to properly interpret the results of predictive analysis and make informed decisions.
Tools and technologies for predictive analysis in energy operations
Predictive analysis requires the use of appropriate tools and technologies. One of the main tools used in the energy sector is machine learning, a technique that allows computers to learn from data and improve their performance over time. Machine learning can be used to build predictive models that can be used to forecast energy demand, optimize energy production, and identify machinery failures in advance. Other tools and technologies used include real-time data analysis, artificial intelligence, and the Internet of Things (IoT).
Challenges and limitations of predictive analysis in the energy sector
Despite the numerous benefits, predictive analysis in the energy sector also presents some challenges and limitations. One of the main challenges is the availability and quality of data. To obtain accurate results, it is necessary to have complete and high-quality historical data, as well as real-time data. Additionally, predictive analysis requires significant computational resources and specialized skills, which may not be readily available to all companies in the energy sector. Finally, predictive analysis can be influenced by unpredictable external factors, such as regulatory changes or fluctuations in commodity prices.
Best practices to maximize the effectiveness of predictive analysis in energy operations
To maximize the effectiveness of predictive analysis in energy operations, it is important to follow some best practices. Firstly, it is essential to clearly define the objectives of predictive analysis and identify the key performance indicators (KPIs) that will be monitored. Secondly, it is necessary to collect and integrate data from various sources, such as sensors, monitoring systems, and historical data. Additionally, it is important to continuously monitor and evaluate the results of predictive analysis to identify any issues or areas for improvement. Finally, it is crucial to engage key personnel and provide the training and support necessary to properly use the results of predictive analysis in daily decision-making.
The future of predictive analysis in the energy sector
The future of predictive analysis in the energy sector looks promising. With continuous advancements in technology and increasing adoption of the Internet of Things, predictive analysis is expected to become increasingly sophisticated and accessible to companies in the energy sector. This will enable them to gain further competitive advantages by improving the efficiency of their operations and reducing costs. Additionally, predictive analysis could also help facilitate the transition to renewable and sustainable energy sources, allowing companies in the energy sector to optimize energy production from sources such as solar and wind.
Predictive analysis offers a tremendous opportunity to revolutionize energy operations, improving the efficiency and profitability of companies in the sector. However, implementing predictive analysis requires a solid IT infrastructure, accurate data collection and integration, and specialized expertise in interpreting the results. By following best practices and addressing associated challenges, companies in the energy sector can maximize the effectiveness of predictive analysis and achieve lasting competitive advantages. The future of predictive analysis in the energy sector looks bright, with the potential to drive the transition to a more sustainable and efficient energy future.